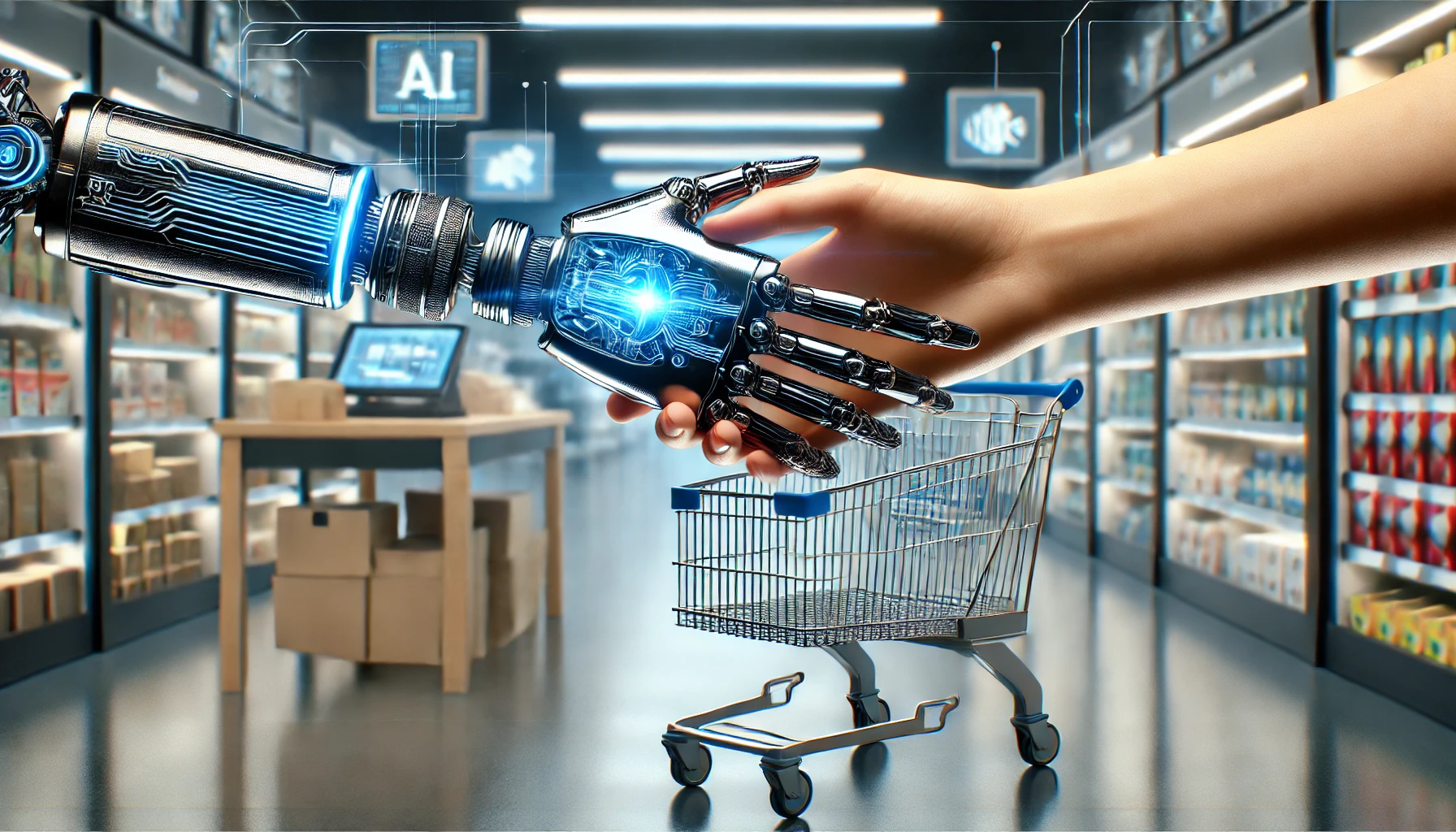
1. Introduction to AI in Retail
Artificial Intelligence (AI) is no longer a futuristic concept; it’s a transformative force shaping the present and future of various industries, including retail. As the retail landscape becomes increasingly competitive and complex, AI is emerging as a critical tool for retailers looking to enhance their operations, streamline processes, and meet the evolving demands of consumers. One of the most significant applications of AI in retail is predictive analytics, particularly in the realm of inventory management. Predictive analytics leverages AI to forecast demand, optimize stock levels, and ultimately, ensure that retailers have the right products in the right place at the right time. This article explores how AI, through predictive analytics, is revolutionizing inventory management in the retail sector.
2. The Role of Predictive Analytics in Retail
Predictive analytics refers to the use of historical data, machine learning algorithms, and statistical techniques to predict future outcomes. In the context of retail, predictive analytics is employed to forecast sales, anticipate demand fluctuations, and optimize inventory management. Unlike traditional methods, which often rely on manual data entry and historical trends, predictive analytics provides real-time insights and forward-looking predictions that are much more accurate and actionable. This approach enables retailers to make informed decisions that improve efficiency, reduce costs, and enhance customer satisfaction.
3. The Evolution of Inventory Management
Inventory management has always been a critical component of retail operations. Traditionally, it involved maintaining sufficient stock levels to meet customer demand while minimizing the costs associated with excess inventory. However, this balancing act has become increasingly difficult as consumer preferences evolve, product lifecycles shorten, and supply chains become more complex. Traditional inventory management methods, such as periodic stocktaking and reorder point calculations, are often too slow and imprecise to keep up with the dynamic nature of modern retail. As a result, retailers have turned to AI and predictive analytics to develop more sophisticated and responsive inventory management systems.
4. How Predictive Analytics Enhances Inventory Management
Predictive analytics enhances inventory management by leveraging vast amounts of data to forecast demand, optimize stock levels, and prevent issues like overstocking and stockouts. By analyzing historical sales data, market trends, seasonal patterns, and even social media activity, AI-driven predictive analytics can accurately predict which products will be in demand and when. This enables retailers to adjust their inventory levels proactively, ensuring they have enough stock to meet customer demand without tying up too much capital in excess inventory.
For example, predictive analytics can help retailers identify slow-moving products and recommend discounts or promotions to clear out inventory before it becomes obsolete. Conversely, it can also identify fast-selling items and prompt retailers to reorder stock before they run out. This level of precision in inventory management not only reduces costs but also improves customer satisfaction by ensuring that products are always available when customers want them.
5. AI Algorithms in Predictive Analytics
The power of predictive analytics in inventory management lies in the AI algorithms that process and analyze data. These algorithms can be broadly categorized into machine learning models, which include supervised, unsupervised, and reinforcement learning. Supervised learning models, for example, are trained on historical data to predict future outcomes, making them ideal for demand forecasting. Unsupervised learning models, on the other hand, can identify patterns and relationships in data without prior knowledge, helping retailers uncover hidden trends and opportunities.
Reinforcement learning, a more advanced type of AI, involves algorithms that learn from their actions over time, refining their predictions and recommendations as they gather more data. This is particularly useful in dynamic retail environments where conditions can change rapidly. By continuously learning and adapting, AI algorithms can provide increasingly accurate predictions, enabling retailers to optimize their inventory management strategies effectively.
6. Demand Forecasting: The Heart of Predictive Analytics
At the core of predictive analytics in inventory management is demand forecasting. Accurate demand forecasting is essential for maintaining the delicate balance between having too much and too little stock. Traditional demand forecasting methods often rely on historical sales data and linear trend analysis, which can be inadequate in today’s fast-paced retail environment. AI-enhanced demand forecasting, however, uses advanced techniques such as time series analysis, regression models, and neural networks to predict future demand with a much higher degree of accuracy.
These AI-driven models consider a wide range of factors, including historical sales, market trends, economic indicators, and even external factors like weather patterns or social media sentiment. By integrating these diverse data sources, AI can generate more accurate and reliable demand forecasts, helping retailers make better decisions about how much stock to order and when.
7. Real-time Data Integration
One of the key advantages of AI-driven predictive analytics is its ability to integrate and analyze real-time data from multiple sources. In retail, this can include sales data, customer behavior data, market trends, and even social media activity. By processing this data in real time, predictive analytics provides retailers with up-to-the-minute insights into what products are selling, where demand is highest, and how inventory levels need to be adjusted.
Real-time data integration also allows retailers to respond quickly to changes in the market. For example, if a sudden spike in demand is detected for a particular product, predictive analytics can alert the retailer to reorder stock immediately, preventing potential stockouts. Similarly, if a product is not selling as expected, the system can recommend promotional strategies to boost sales or reduce inventory.
8. Benefits of AI-Driven Inventory Management
The implementation of AI-driven predictive analytics in inventory management offers numerous benefits for retailers. Firstly, it leads to significant cost savings by reducing the need for excess inventory and minimizing stockouts. By accurately forecasting demand and optimizing stock levels, retailers can avoid the costs associated with overstocking, such as storage fees and markdowns, as well as the lost sales opportunities caused by stockouts.
Secondly, AI-driven inventory management enhances customer satisfaction. When products are consistently available, customers are more likely to have a positive shopping experience, which can lead to increased loyalty and repeat business. Moreover, by optimizing inventory levels, retailers can offer a wider range of products without the risk of overstocking, further improving the customer experience.
Additionally, AI-driven inventory management contributes to sustainability by reducing waste. Overstocked products that go unsold often end up being discarded, which is both costly and environmentally harmful. By minimizing excess inventory, retailers can reduce waste and their overall environmental impact.
9. Challenges and Limitations of AI in Inventory Management
Despite its many advantages, the implementation of AI-driven predictive analytics in inventory management is not without challenges. One of the primary concerns is data privacy. As AI systems require access to large amounts of data to function effectively, retailers must ensure that they are collecting, storing, and using customer data in a way that complies with privacy regulations and protects consumer rights.
Another challenge is the cost and complexity of implementing AI systems. While large retailers may have the resources to invest in advanced AI tools and technologies, smaller retailers may find it more difficult to justify the expense. Furthermore, the effectiveness of AI in inventory management depends on the quality of the data being used. Inaccurate or incomplete data can lead to incorrect predictions and suboptimal decisions.
Finally, there is the issue of balancing automation with human oversight. While AI can greatly enhance inventory management, it is important for retailers to maintain a level of human oversight to ensure that decisions are aligned with the company’s overall strategy and objectives. Retailers must also be prepared to intervene when AI-driven systems encounter unexpected situations or make recommendations that are not practical or feasible.
10. Real-World Examples of AI in Retail Inventory Management
Several leading retailers have successfully implemented AI-driven predictive analytics to optimize their inventory management. For example, Walmart uses AI to analyze vast amounts of data from its global supply chain to predict demand and manage inventory levels across its stores. This has enabled Walmart to reduce stockouts, improve product availability, and increase overall efficiency.
Amazon is another example of a retailer that has leveraged AI to revolutionize inventory management. Through its advanced AI-driven systems, Amazon can forecast demand, optimize stock levels, and manage its vast inventory with unparalleled precision. This has allowed the company to offer a wide range of products with fast delivery times, contributing to its dominance in the e-commerce space.
Even smaller retailers are beginning to adopt AI-driven inventory management systems. For instance, online fashion retailer Stitch Fix uses AI to predict customer preferences and manage inventory accordingly. By analyzing customer data, the company can ensure that it has the right mix of products in stock to meet demand, leading to higher customer satisfaction and reduced inventory costs.
11. The Future of AI and Predictive Analytics in Retail
The future of AI in retail, particularly in inventory management, looks promising. As AI technology continues to evolve, we can expect to see even more advanced predictive analytics tools that offer greater accuracy and deeper insights. For example, emerging technologies such as quantum computing and advanced neural networks could further enhance the capabilities of AI-driven predictive analytics, allowing retailers to make even more precise and informed decisions.
Moreover, the post-pandemic retail landscape is likely to accelerate the adoption of AI. As retailers look for ways to navigate the challenges posed by changing consumer behavior, supply chain disruptions, and economic uncertainty, AI-driven predictive analytics will play a crucial role in helping them adapt and thrive.
In the coming years, we can expect to see AI become even more integrated into retail operations, from inventory management to customer service. Retailers that embrace these technologies will be better positioned to meet the demands of the modern consumer and succeed in an increasingly competitive market.
12. How Retailers Can Implement AI for Inventory Management
For retailers looking to implement AI-driven predictive analytics for inventory management, the first step is to identify the specific needs and goals of their business. This includes assessing current inventory management practices, identifying pain points, and determining how AI can address these challenges.
Next, retailers should choose the right AI tools and platforms that align with their business needs. This may involve partnering with AI vendors or developing in-house solutions, depending on the retailer’s resources and capabilities.
Training staff is also a critical component of successful AI implementation. Retailers must ensure that their employees are equipped with the knowledge and skills needed to work with AI-driven systems and make data-driven decisions. This may involve providing training programs, workshops, or hiring experts in AI and data science.
Finally, retailers should approach the transition to AI with a mindset of continuous improvement. As AI systems learn and evolve over time, retailers must be prepared to refine and adjust their inventory management strategies to maximize the benefits of predictive analytics.
13. Ethical Considerations in AI-Driven Retail
As AI becomes more prevalent in retail, it is essential to consider the ethical implications of these technologies. One of the key concerns is ensuring transparency and fairness in AI-driven decision-making. Retailers must be transparent about how they use AI and ensure that their systems are free from biases that could lead to unfair treatment of customers or employees.
Another ethical consideration is the impact of AI on employment. While AI-driven inventory management can lead to greater efficiency and cost savings, it may also result in job displacement, particularly for roles traditionally involved in inventory management and logistics. Retailers must carefully consider the social impact of AI and explore ways to mitigate any negative effects, such as reskilling programs for affected employees.
Finally, it is important to address the potential for bias in AI algorithms. Since AI systems learn from historical data, there is a risk that they may perpetuate existing biases or create new ones. Retailers must ensure that their AI systems are designed and monitored to detect and correct biases, ensuring fair and equitable outcomes for all stakeholders.
14. Measuring the Success of AI in Inventory Management
To determine the success of AI-driven inventory management, retailers should track key performance indicators (KPIs) that align with their business goals. These KPIs may include inventory turnover rates, stockout occurrences, carrying costs, and customer satisfaction levels.
Retailers should also consider the long-term benefits of AI, such as improved decision-making, enhanced operational efficiency, and increased competitiveness. While the initial costs of implementing AI may be high, the long-term returns in terms of cost savings, customer satisfaction, and business growth can be significant.
Continuous improvement is another important aspect of measuring success. Retailers should regularly review and refine their AI-driven inventory management strategies, using data and insights to make adjustments and optimize performance over time.
15. Conclusion: The Transformation of Retail Through AI
The integration of AI and predictive analytics into inventory management is revolutionizing the retail industry. By providing retailers with the tools to forecast demand, optimize stock levels, and make data-driven decisions, AI is helping to create more efficient, responsive, and customer-centric retail operations. As AI technology continues to advance, its role in retail is set to grow even further, offering new opportunities for innovation and growth. Retailers that embrace AI-driven inventory management will be well-positioned to meet the challenges of the modern retail landscape and succeed in an increasingly competitive market.